Multi-length scale scanning X-ray imaging/tomography workflow
The workflow implemented at Nanoscopium for multi-length scale and multimodal scanning X-ray imaging and tomography experiments is presented in Fig. 1: the sample mounting and alignment are followed by sparse tomography of the whole sample, and then the visualization of the volume rendering of the reconstructed tomograms. The strategy of high-resolution (HR) measurements is based on these medium-resolution results. This workflow permits even users who are new to X-ray imaging and tomography, to accomplish all measurement and data reconstruction steps during their experiment. At Nanoscopium all user projects apply for XRF multi-scale imaging or tomography, which is often complemented by other modalities (absorption- or phase-contrast imaging/tomography, XANES, or XRD) to best tackle the actual scientific question. As such, in this paper, we have chosen scanning XRF tomography to demonstrate our approach.
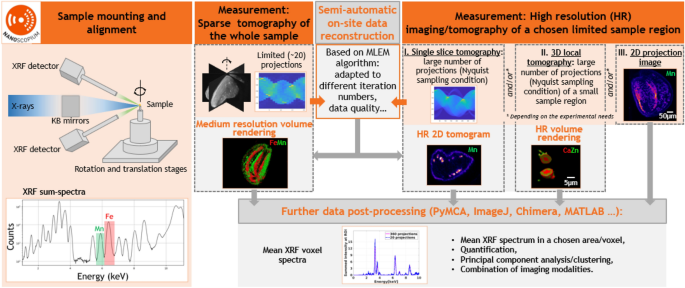
Semi-automatic multi-scale XRF and multimodal scanning imaging and tomography workflow implemented at Nanoscopium. After sample mounting and alignment, sparse scanning tomography is performed on the whole sample. Some minutes after the experiment, medium resolution volume rendering is available for data interpretation by the proposed semi-automatic on-site data reconstruction. This permits optimizing the strategy of successive high-resolution (HR) measurements. The on-site reconstruction algorithm provides HR tomograms for data interpretation and further data processing some minutes after the HR experiments. The measurement and data reconstruction steps are included in orange and grey rectangles, respectively.
As a first step, a sparse XRF/multimodal tomography measurement with 20 projection angles (see details in “Data acquisition” section) is followed by semi-automatic on-site tomography reconstruction. Direct visualization of a specimen’s reconstructed medium-resolution 3D elemental distribution and morphology enables immediate identification of information pertinent to the research project. This permits users to choose the strategy for succeeding in high-resolution (HR) measurements for studying the smallest sample features crucial to tackling the scientific problem32. This HR measurement can be 2D projection imaging, 2D single slice tomography, local scanning 3D tomography, or any combination of these. Notably, a reasonable trade-off must be made during a tomography experiment regarding acquisition time, spatial resolution, and the number of samples wished to be investigated. After the high-resolution experiment, an estimate of the spatial resolution achievable by different projection numbers can be obtained by the Fourier ring correlation (FRC) calculation (detailed in the “Methods” section). If the resolution of the first sparse tomography results does not meet the user’s requirements, then a second sparse tomography measurement can be performed. This will start with an angular offset equal to the half angular step of the first sparse tomography experiment. The second optional sparse tomography, having the same projection numbers as the first, results in doubling the total number of projections and hence improving the spatial resolution. As demonstrated in Fig. 1, all these modalities can be reconstructed semi-automatically on-site during the experiment using predefined and pre-parameterized reconstruction algorithms with no need for interaction from the users. Hence, users can guide their experiments and redefine the scientific objectives on-the-fly depending on the results obtained on-site.
Moreover, the HDF5 data format of the reconstructed tomograms is fully compatible with widely used cross-platform freewares and open-source data analysis tools, such as PyMCA (XRF data processing), ImageJ, Chimera (imaging/tomography data processing), etc. As such, further on-site or post-experiment processing, such as extraction of mean XRF spectra, quantification, combined treatment of different imaging modalities, and multivariate statistical analysis (Principal Component Analysis, Cluster Analysis, etc.)33, can be obtained straightforwardly in any 2D/3D feature identified from the projection images or the reconstructed 2D/3D tomograms.
Proof of principle test measurements: Wild-type Arabidopsis thaliana seeds
Arabidopsis thaliana is a weed native to Eurasia and Africa with a short life cycle (≥ 6 weeks). This annual plant is a popular model organism in plant biology due to the knowledge of its genome and the availability of numerous mutants. The wild type (Col-0) is commonly used in plant biological laboratory experiments and even in Space experiments to study genetics, evolution, and development of flowering plants. As a model plant, Arabidopsis thaliana is also a powerful tool to investigate metal homeostasis and nutrient distribution, relevant questions about the world’s food production and the agriculture industry. In this context, essential elements Fe and Mn in the plant seed are crucial for plant germination. Mn, involved in the photosystem II in chloroplasts, is needed for the vigour of germinating plants, and Fe is involved in various metabolic processes (respiration, photosynthesis…). These two elements have different physiological functions and different transporter pathways. Thus, differences are expected in their distribution and concentration within the seed. 2D XRF imaging is a powerful tool for locating trace elements in plants. However, assigning the measured metal distribution unambiguously to the grain’s ultrastructure can be challenging due to the several hundreds of microns information depth of Mn and Fe. Moreover, sample sectioning, required to study the internal elemental distribution by 2D scanning XRF imaging, can be intricate for small and hard samples such as A. thaliana seeds and can induce tissue alteration and artifacts.
XRF and multimodal tomography are useful complementary tools for determining metal concentrations and distribution in intact seeds with minimal sample preparation16,34,35.
Sparse scanning X-ray tomography of a whole seed
We tested the performance of our tomography approach on wild-type Arabidopsis thaliana seeds. One advantage of using seeds, in general, is their low water content, resulting in lower amounts of radiation damage than more hydrated tissues. In order to obtain an overview of the elemental distribution of a whole mesoscale seed of 700 µm dimensions, we performed simultaneous sparse XRF (see Fig. 2) and absorption (Fig. S1) micro-tomography. The figure of merit of measuring a limited number of projections is to identify, within acceptable measurement times, the features of interest, in our case the distribution of Mn and Fe within different seed compartments. Since the total measurement time of a tomography experiment scales proportionally with the number of projections, it can be drastically reduced by measuring only a few angular projections. This also reduces the eventual radiation damage of the sample, which might be important in the case of scanning imaging of larger samples. However, the reduced number of projections should be chosen without significantly compromising the quality of the obtained 3D mesoscale tomograms. The reconstructed sparse 3D tomograms of Mn and Fe can be seen in Fig. 2A, B. It comprises 223 virtual slices and is calculated from 20 measured angular projections by the maximum-likelihood expectation–maximization (MLEM) algorithm (described in “Methods” section). It is clear from the reconstructed sparse tomograms that Fe is preferentially located in the provascular systems of the seed, and that Mn is mainly distributed in the abaxial area of both cotyledons, at the subepidermal level, as well as in the cortex area of the hypocotyl. These observations are in agreement with the results published in the literature34,35.
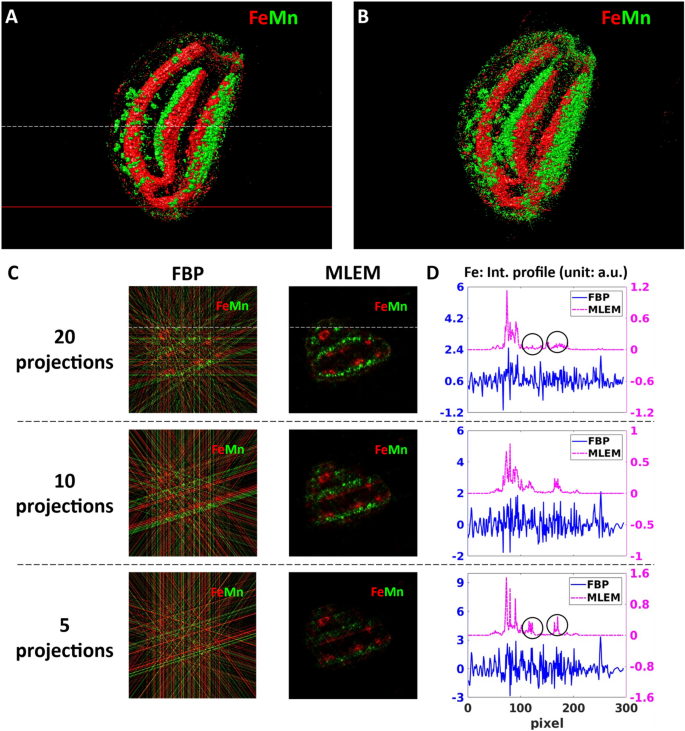
Reconstructed sparse 3D Fe and Mn tomograms and a virtual slice showing the internal Fe and Mn distributions. (A,B) volume rendering of the Fe and Mn tomograms reconstructed by MLEM from 20 (A) and 5 angular projections (B). The white dashed line indicates the altitude of the virtual slice shown in (C). The red line marks the altitude of the high-resolution single slice tomography shown in Fig. 3. (C) Comparison of the reconstructed results obtained by the FBP and MLEM algorithms in the function of the measured number of angular projections. The columns correspond to two different algorithms: filtered back-projection (FBP) and maximum likelihood expectation maximization (MLEM). The lines correspond to 20, 10, and 5 angular projections, respectively. (D) Comparison of the intensity profiles of Fe obtained by FBP and MLEM. The Fe intensity profiles were extracted along the white straight line indicated in (C).
To optimize the measurement conditions and the corresponding reconstruction parameters of sparse tomography, we compared two different reconstruction algorithms, Filtered back- projection (FBP) and MLEM, in the function of the number of measured angular projections (5, 10, and 20). The comparison is demonstrated in Fig. 2C, D using the virtual slice marked by a white dashed line in Fig. 2A.
FBP algorithm is the standard solution for 3D tomography reconstruction due to its fast reconstruction time and easy implementation. However, because of the intrinsic nature of the algorithm, FBP has severe limitations in the case of noisy datasets and highly under-determined measurement conditions with a small number of projections36. This can be clearly seen in the first column in Fig. 2C, where the signal-to-noise ratio of all sparse tomograms obtained from 5, 10, and 20 projections is very low. Indeed, the strong streak artifacts of FBP, caused by the small number of projections, shadow the elemental distributions: the Mn and Fe distributions are hardly visible even in the case of 20 angular projections. In effect, as shown by the Fe intensity profiles (shown in Fig. 2D by the blue curves), these strong artifacts, resulting also in negative intensity values, are hiding the information on the Fe variation within the sample. As such, since FBP cannot significantly reduce streak artifacts at low number of projections, it is not adapted to sparse tomography reconstruction.
MLEM algorithm (second column of Fig. 2C) is superior to FBP in handling noisy datasets. Moreover, it includes the non-negativity constraint assumption. In our workflow, we included a simple automatic stopping criterion for the MLEM algorithm with the smallest possible noise as a figure of merit (as detailed in “Methods” section). This choice results in terminating the reconstruction process at small number of iterations (thus with short reconstruction time) introducing only a few, weak artifacts in the reconstructed tomograms. The reconstructed tomograms in Fig. 2C illustrate the robustness of this algorithm in treating limited number of projections. Moreover, with increasing number of projections the reconstruction artifacts of MLEM are becoming weaker. This is illustrated by the purple Fe profiles in Fig. 2D, where the strongest reconstruction artifacts marked by the black circles in the 5-projection tomogram (last line in D) diminished in the 20-projection tomogram (first line of D).
High-resolution single slice tomography
The above-described 3D sparse tomography permits choosing the best angular position for high-resolution 2D projection imaging, as shown in Fig. 3A for Mn distribution. It also allows choosing the altitude(s) for high-resolution single slice tomography (Fig. 3B, C). This provides insight into the internal Mn and Fe distributions of subcellular features within intact seeds. We performed HR single slice XRF tomography at the altitude shown by the red line in Fig. 2A. The FBP and MLEM reconstructions of the internal 2D Mn distribution can be seen in Fig. 3B, C. We included for comparison the sparse tomogram of Mn obtained by MLEM in the very same virtual slice (Fig. 3D). The reconstructed features are in good agreement between the sparse (Fig. 3D) and high-resolution tomography (Fig. 3B, C) results. However, as expected, high-resolution tomography reveals fine details with improved spatial resolution, which are non-detectable or hardly identifiable by sparse tomography. For such HR tomography, the use of FBP (Fig. 3B) is straightforward and is the fastest reconstruction algorithm, where the computation time is proportional to the registered number of projections. However, even with 360 angular positions, there are non-negligible streak artifacts due to insufficient angular projections compared to the number of scanned pixels in this mesoscale sample (see Nyquist sampling condition in Eq. 3). The reconstruction result of the MLEM algorithm (Fig. 3C) provides better contrast. Moreover, the computation time of < 2 s/sinogram is significantly faster than the measurement time of some hours (and will be comparable with the measurement time of some minutes/sparse tomography at a 4th generation synchrotron). As such, the MLEM algorithm proved to be the best compromise for semi-automatic image reconstruction for both 3D sparse tomography and 2D high-resolution single slice tomography experiments.
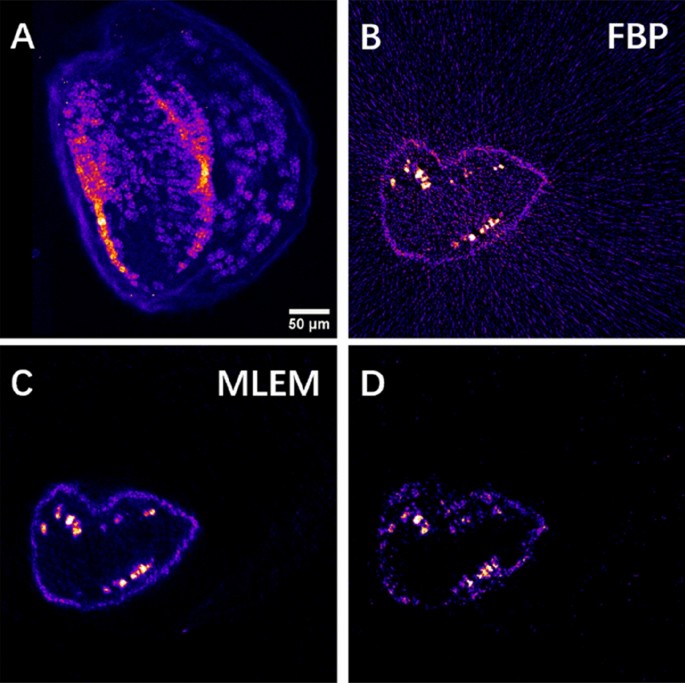
High-resolution 2D scanning XRF imaging and tomography of the Arabidopsis seed. (A) High-resolution 2D projection image of the Mn distribution at an appropriate angle chosen from the 3D sparse tomograms. (B,C) Reconstructed HR single slice of the Mn tomogram obtained by the FBP algorithm (B) and by the MLEM algorithm (C) measured at the altitude marked by the red line in Fig. 2A. (D) Mn sparse tomography reconstruction at the same slice obtained by MLEM.
Spatial resolution of the tomograms reconstructed by MLEM
To estimate the spatial resolution of the tomograms reconstructed by the MLEM algorithm, we extracted two subsets with uniform angular sampling from the 2D high-resolution dataset. Taking the 2D high-resolution sinogram of 360 projections as an example, the full projection dataset was divided into two subsets, both having the same projection numbers. These two subsets were reconstructed independently by MLEM with the automatically determined iteration numbers. The two independent tomograms were then used for FRC analysis.
Figure 4 shows the FRC curves between the two tomograms reconstructed using a different number of projections from the same 2D high-resolution sinogram. The spatial resolution was determined at the intersection of the FRC curve and the fixed 0.5 threshold37. Table 1 shows the spatial resolution determined by the 0.5 threshold criterion in the function of the number of projections. The spatial resolution improves with the number of projections as expected (see Figs. S1, C and E). A resolution estimate of 8.4 μm was obtained for the 20-projection tomogram. The MLEM iterative method has resulted in a significant resolution improvement compared to the spatial resolution given by the Nyquist sampling condition.
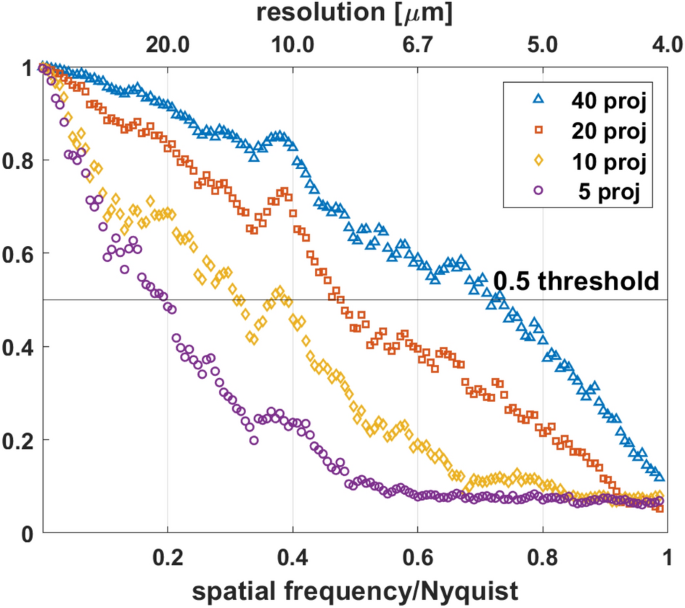
Spatial resolution estimation by Fourier ring correlation (FRC) method. Estimation of spatial resolution for tomograms reconstructed by MLEM from different numbers of projections chosen from the measured 360 angular projection dataset. The fixed 0.5 threshold was used for FRC analysis. The Nyquist frequency is 0.25 μm−1.
Renal papilla sample
In order to test the performance of our workflow in missing wedge measurement conditions and for HR 3D local tomography, we investigated mouse renal papilla samples. Nowadays, the formation of renal stones affects 10% of the global population38. Most of the stones develop on Randall’s Plaque, a mineral deposit at the tip of renal papillae39. The major components of Randall’s Plaque are calcium phosphate apatite and amorphous carbonated calcium phosphate40. Therefore, the distribution of Ca indicates the position of calcification in the renal papilla sample. Studying the correlation and colocalization between Ca and other major and trace components permits to reveal those trace elements, that are involved in early-stage calcification processes. Understanding their role in the pathological process opens the way toward efficient prevention and treatment of the renal stone formation. The sample we used in the present study is a mouse papilla affected by calcium phosphate deposits similar to the human Randall’s plaque (see41 for more details on the sample preparation).
Sparse and high-resolution XRF tomography of renal papilla
The semi-automatic workflow has been used to study the elemental abundancies within 50 µm thick slices of renal papilla samples (Fig. 5A, B). In this case, the fixation of the thin biological sample of ~ 500 µm lateral dimensions on a Si3N4 membrane caused a missing wedge of 2 × 28° in the tomography measurement. This poses a specific challenge to tomographic reconstruction42. This was successfully tackled by the MLEM algorithm, which proved to be the best compromise also for missing wedge tomography. 3D sparse tomography performed on the top of the sample showed substantial colocalizations between Zn and Ca (Fig. 5C) within dense sample regions revealed by simultaneous transmission imaging (Fig. 5B). However, the ~ 8.5 µm medium spatial resolution of sparse tomography does not permit to reveal the details of micrometer-sized Ca- and Zn-rich features, that are related to early-stage calcification process. Gaining insight, with high spatial resolution, into the elemental distribution of these micrometer-sized Ca and Zn containing spherical structures is crucial. We performed 3D local tomography around a chosen micro-sphere (red rectangles in Fig. 5B, C). The angular projection images were measured with 500 nm pixel size. Figure 5D, E show the volume rendering of Zn and Ca within the measured micrometer-scale calcification sphere. In the cut-off view of the 3D volume rendering in Fig. 5E the internal distribution of Zn and Ca is shown within the ~ 10 µm dimension calcification sphere. These results reveal that Zn is enriched within a few micrometers thick rim on the surface of the calcification micro-sphere. Since Zn is considered to be a marker of inflammation43, this result also indicates that the calcium phosphate deposition in the medullary interstitial is a pathological process.
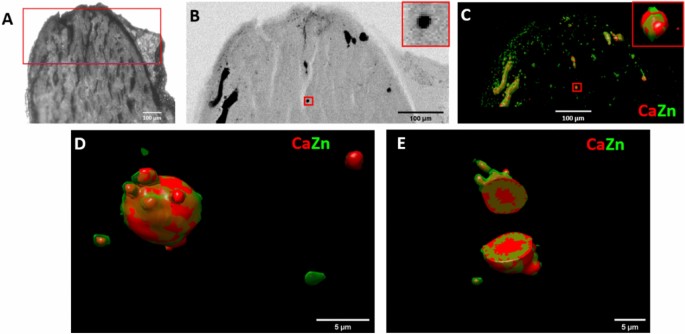
Sparse and local scanning X-ray tomography of a renal papilla sample. (A) Optical microscope image of the mouse papilla sample, mimicking human kidney calcifications. The scanned region is marked in red. (B) Transmission image of the tip of the sample. The small red rectangle in the middle marks the sample region chosen for local tomography. The zoom-in image of this region is inserted into the upper-right corner. (C) Sparse tomography reconstruction of Zn and Ca. The small red rectangle in the middle marks the sample region chosen for local tomography. The zoom-in image of this region is inserted into the upper-right corner. (D) Reconstructed 3D local tomogram of Ca and Zn of the calcification micro-sphere marked by the red rectangles in (B,C). (E) Cut-off view at the middle of the calcification micro-sphere presented in (D).
Mean XRF voxel-spectrum
Next to the elemental distribution maps, complete local XRF spectral information is necessary to obtain detailed information on the chemical composition of local features. This might reveal rare characteristics appearing only in particular sample locations/voxels (“needle in the haystack problem”). Since we collect the full XRF spectrum in each measured pixel during data collection, next to the tomograms of the predefined elements, it is also possible to reconstruct the full XRF spectrum in each voxel of the tomogram. In other words, we can include a spectral dimension to the reconstructed dataset, which can be used to extract the mean XRF spectrum of any chosen sample area or volume. Such complete hyperspectral tomography reconstruction was realized for each energy channel of the measured XRF spectra. At 10 keV excitation energy, this results in 1000 sinograms for each virtual slice, and the reconstruction process, even with the MLEM iterative method and multi-core, is becoming quite time-consuming. For example, the reconstruction of the high-resolution 3D hyperspectral XRF tomogram (with the energy as the 3rd dimension) took ~ 1 h by MLEM for the Arabidopsis seed.
Figure 6A shows the virtual slice of the Arabidopsis seed reconstructed from the sum-XRF spectra of the dataset containing 360 projections. The mean XRF spectra extracted from the area marked by a red circle in Fig. 6A by FBP and MLEM can be seen in Fig. 6B, C. The mean XRF spectra of the chosen region obtained by FBP and MLEM do not show a significant difference; the total intensities of the XRF spectra agree within 5%. As such, in the case of high-resolution tomography, FBP is a good compromise to obtain local spectral information within a limited reconstruction time.
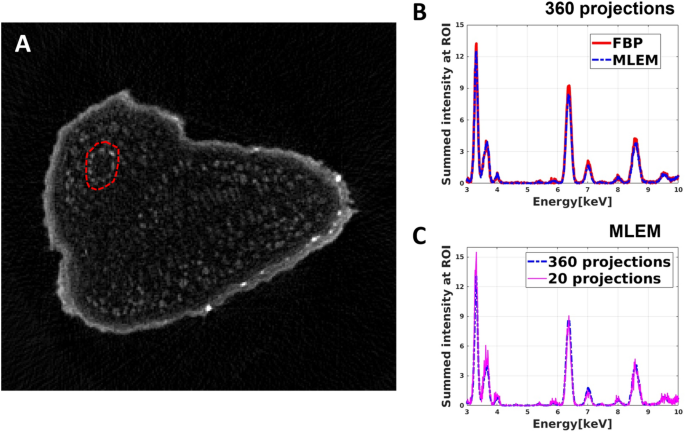
Extraction of the mean XRF spectra of a chosen area in a virtual slice of the Arabidopsis seed. (A) Reconstructed high-resolution virtual slice of the sum XRF spectra of the Arabidopsis seed. (B) Comparison of the mean XRF spectra of the selected area, marked by a red dashed circle in (A), obtained by FBP (red curve) and MLEM (blue curve) algorithm. (C) The mean XRF spectra of the selected area obtained by MLEM from 360 projections (blue curve) and from 20 projections (fuchsine curve).
As the next step, we selected equiangularly 20 projections out of the 360 ones to simulate a sparse tomography in identical experimental conditions. This allows comparing the mean XRF spectra obtained by sparse and high-resolution tomographies. In the case of sparse tomography, FBP cannot be used for data reconstruction due to the low number of projections. As such, we reconstructed the sparse tomogram for each energy channel by MLEM. The mean XRF spectrum of the same region as before (red circle in Fig. 6A) was calculated from this sparse tomography dataset. Figure 6C shows that the two mean XRF spectra agree well, which illustrates that the MLEM algorithm provides reliable hyperspectral tomograms and mean XRF spectra even for a severely limited number of projections.
The mean XRF spectrum within a selected volume of interest of the calcification sphere (shown in Fig. 5D) of the renal papilla is demonstrated in Fig. 7. The reconstruction process for the 4D hyperspectral tomography dataset (with the energy as the 4th dimension) took around 1 h by the MLEM algorithm. To obtain the mean XRF spectra within a volume of interest, we selected the 3D volumes of interest by ImageJ, a freeware frequently used by our users. We applied this mask to each energy channel in the reconstructed 4D dataset. The extracted mean XRF spectra shown in Fig. 7B reveal that Ca is the dominant element in the core of the calcification micro-sphere (red XRF spectrum), which also contains Zn. Within the surface rim (indicated by green in Fig. 7A and green XRF spectrum in Fig. 7B), the increased Zn to Ca ratio, due to the threefold larger Zn and ~ 20% smaller Ca content, provides evidence of the association of Zn to the pathological process.
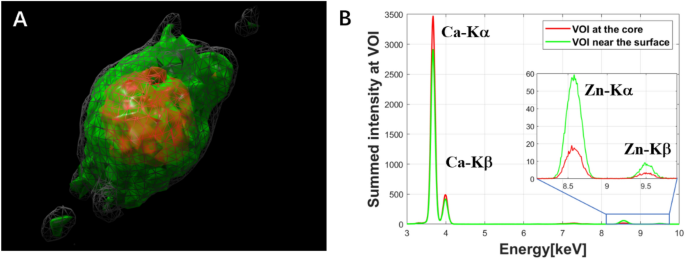
Extraction of the mean XRF spectra within the volume of interest of the reconstructed calcification micro-sphere shown in Fig. 5D,E. (A) Reconstructed result by MLEM algorithm from the total intensity of the XRF spectra, grey volume: reconstructed total sphere volume, red and green volumes: core and surface rim regions, respectively. (B) Comparison of the mean XRF spectra of the core (red) and surface rim (green) volumes.
#routine #characterization #intact #mesoscale #samples #multiscale #multimodal #scanning #Xray #tomography #Scientific #Reports